```
---
## Functions returned by functions
* This is because they were created in different executions of `fboxcox()`
* Although not immediately apparent, these environments retain the value of `lambda`
```r
ls.str(environment(boxcox.sqrt))
```
```
f : function (x)
lambda : num 0.5
```
```r
ls.str(environment(boxcox.log))
```
```
f : function (x)
lambda : num 0
```
---
## Functions returned by functions
* Without lexical scoping, this would not have been possible
* Another example from [The R Paper](https://www.stat.auckland.ac.nz/~ihaka/downloads/R-paper.pdf):
```r
y <- 123
f <- function(x) {
y <- x * x
g <- function() print(y)
g()
}
f(10)
```
```
[1] 100
```
* S, which is similar to R but does not have lexical scoping, would print `123`
---
## A more serious example
* Suppose we want to transform (positive-valued) data $x_1, x_2, \dotsc, x_n$
--
* ... to look as Normal as possible, but we don't know what the correct
transformation should be
* A standard textbook solution is to look for an "optimum" choice within the Box-Cox family
* A maximum-likelihood approach: assume $\lambda$-transformed data follow $N(\mu, \sigma^2)$
--
* A [little mathematics](https://www.isid.ac.in/~deepayan/RT2018/notes/systematic-violations.html#/the-box-cox-transformation-a-likelihood-based-approach) tells us that
- There is no closed form solution for $\lambda$, but
- The optimum (MLE) $\lambda$ can be found by minimizing
$$
f(\lambda) = \frac{n}{2} \left( \log 2 \pi + \log \hat{\sigma}^2(\lambda) + 1 \right) - (\lambda - 1) \sum \log \sub{x}{i}
$$
- where $\hat{\sigma}^2(\lambda)$ is the sample variance of the transformed data
--
* Here $f$ is a function, which is itself a function of data $x_1, x_2, \dotsc, x_n$
---
layout: true
## A quick prototype
---
* Suppose we have a general numerical optimizer that can optimize a function of _one_ argument
* Make a function that converts data into a function suitable for optimization:
```r
negllBoxCox <- function(x)
{
n <- length(x)
slx <- sum(log(x)) # compute only once
function(lambda) {
y <- fboxcox(lambda)(x) # Note use of fboxcox() defined earlier
sy <- mean((y - mean(y))^2)
n * log(sy) / 2 - (lambda - 1) * slx # ignoring constant terms
}
}
```
---
* Let's compute the "negative log likelihood" function for some simulated data
```r
x <- rlnorm(100) # log-normal, so true lambda = 0
f <- negllBoxCox(x)
f(0)
```
```
[1] 4.311438
```
* We can can now use this function in any optimizer
```r
optimize(f, lower = -10, upper = 10)
```
```
$minimum
[1] -0.1608655
$objective
[1] 2.230694
```
```r
## OR optim(par = 1, fn = f) for alternative methods
```
---
* We can even plot $f$ — but first we have to "vectorize" our function
* The `Vectorize()` function creates a vectorized function from a non-vectorized one
```r
plot(Vectorize(f), from = -2, to = 2, n = 1000, las = 1)
```
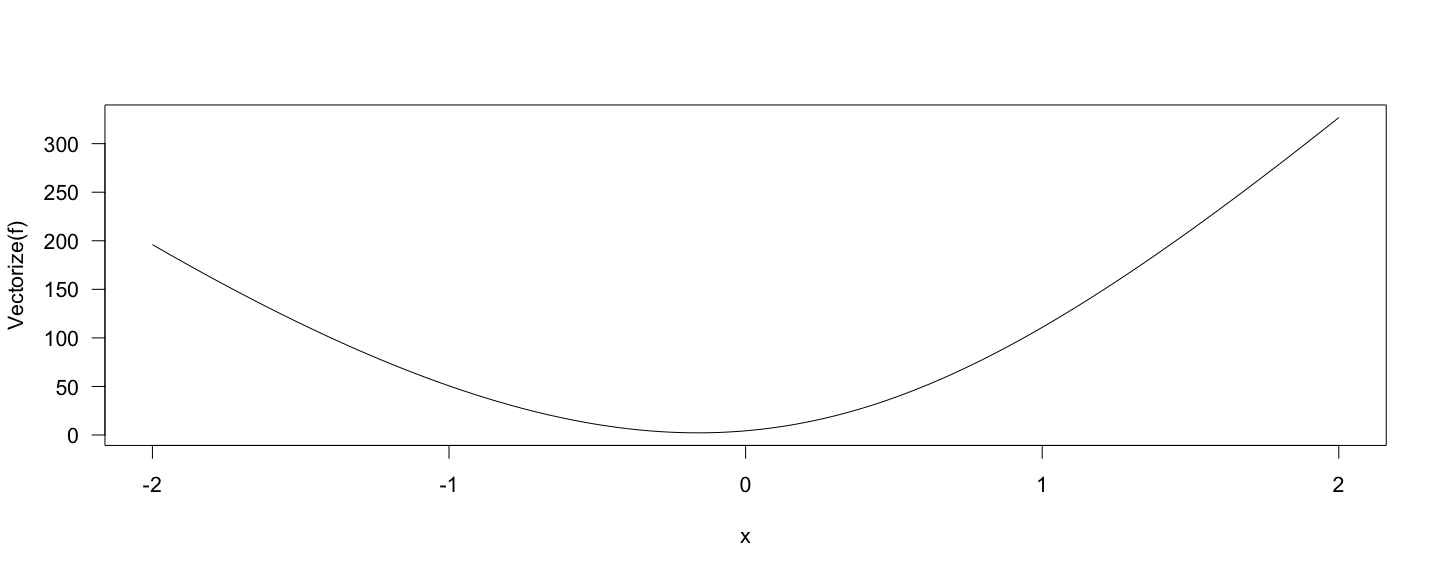
---
* We can also do a quick simulation study of how well this method estimates $\lambda$ when the true $\lambda = 1$
```r
lambda.hat <-
replicate(1000,
optimize(negllBoxCox(rnorm(100, mean = 10)), lower = -10, upper = 10)$minimum)
hist(lambda.hat, breaks = b, las = 1)
```
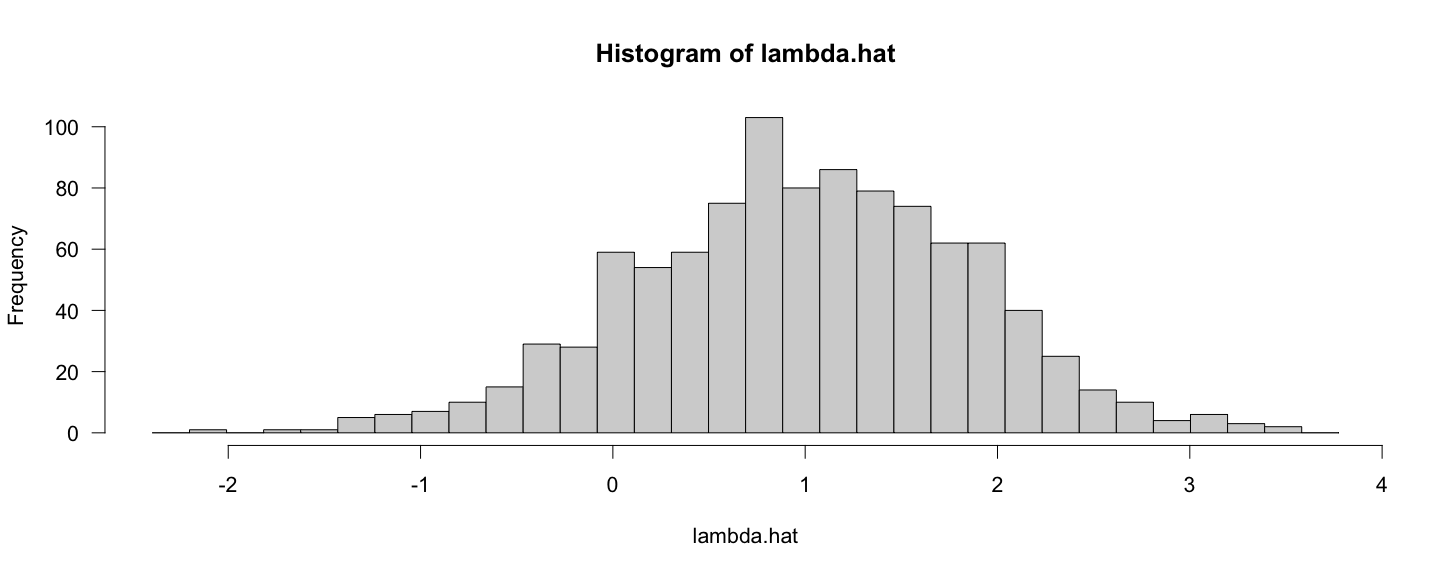
---
layout: false
## Another example: linear regression with general loss function
* Another common use case: optimization of a loss function
* Consider the following general approach to linear regression given data $(\sub{x}{i}, \sub{y}{i}), i = 1,\dotsc,n$
$$
\hat{\beta} = \arg \min_{\beta} \sumlimits{i=1}{n} L(\sub{y}{i} - \sub{\beta}{0} - \sub{\beta}{1} \sub{x}{i}) =
\arg \min_{\beta} f(\beta)
$$
* Common choices for $L$ are
* $L(u) = u^2$
* $L(u) = | u |$
* $L(u) = \begin{cases}
u^2 & \lvert u \rvert \leq c \\
c (2 | u | - c) & \text{otherwise}
\end{cases}$
---
layout: true
## Objective functions (to be optimized)
---
* The following functions takes data and $L$, and creates a suitable objective function
```r
make.objective <- function(x, y, L = abs)
{
function(beta)
{
sum(L(y - beta[1] - beta[2] * x))
}
}
data(phones, package = "MASS")
obj.lad <- with(phones, make.objective(year, calls, L = abs))
obj.lse <- with(phones, make.objective(year, calls, L = function(u) u*u))
```
---
```r
obj.lad
```
```
function(beta)
{
sum(L(y - beta[1] - beta[2] * x))
}
```
```r
environment(obj.lse)$L
```
```
function(u) u*u
```
```r
environment(obj.lad)$L
```
```
function (x) .Primitive("abs")
```
---
layout: true
## Results of optimization
---
```r
fm.lad <- optim(obj.lad, par = c(0,0))
fm.lse <- optim(obj.lse, par = c(0,0))
str(fm.lad)
```
```
List of 5
$ par : num [1:2] -66.05 1.35
$ value : num 844
$ counts : Named int [1:2] 117 NA
..- attr(*, "names")= chr [1:2] "function" "gradient"
$ convergence: int 0
$ message : NULL
```
```r
str(fm.lse)
```
```
List of 5
$ par : num [1:2] -260.12 5.04
$ value : num 69544
$ counts : Named int [1:2] 109 NA
..- attr(*, "names")= chr [1:2] "function" "gradient"
$ convergence: int 0
$ message : NULL
```
---
```r
plot(calls ~ year, phones)
abline(fm.lad$par, col = "blue")
abline(fm.lse$par, col = "red") # This is what we would get from lm()
```
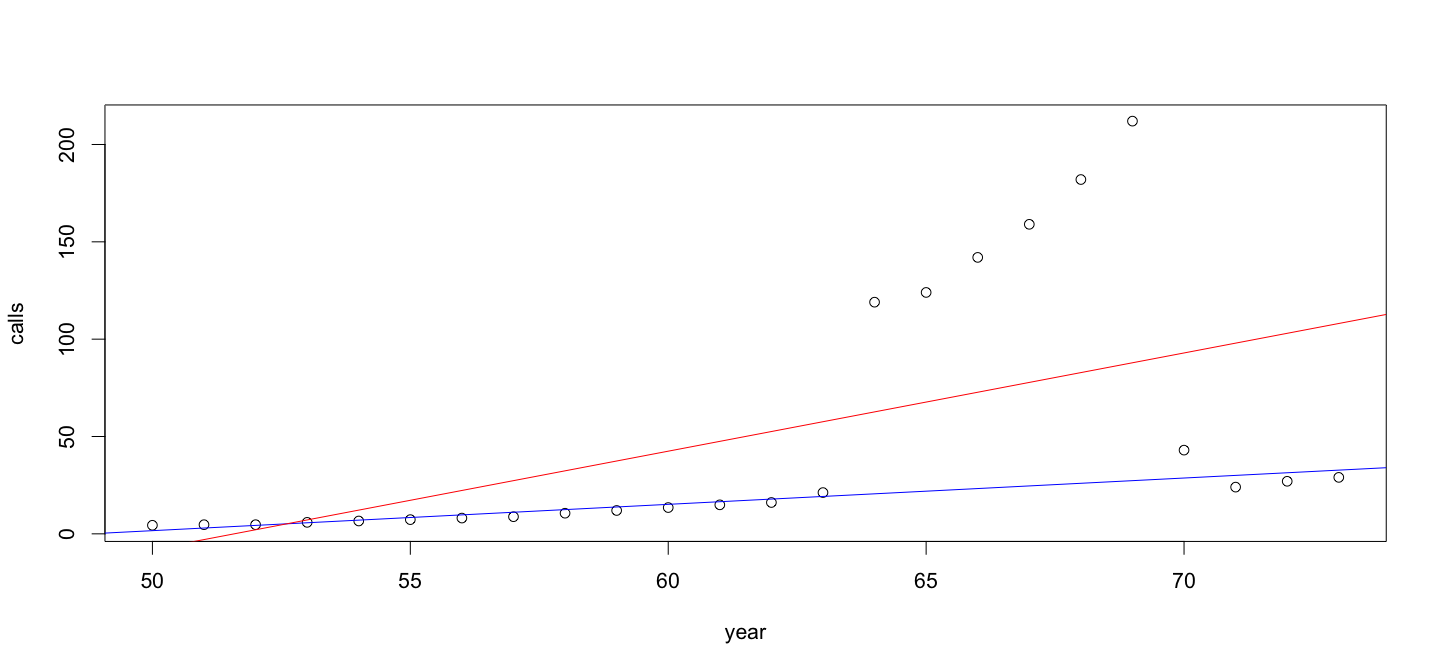
---
layout: false
## A more complex example
* Suppose we want to plot a histogram
* One important parameter is the bin width $h$
* Fixing starting point, what is an optimal choice?
* Maximum Likelihood fails because it "oversmooths", so needs regularization
* Options
* [AIC](https://en.wikipedia.org/wiki/Akaike_information_criterion)
* [cross validation](http://onlinestatbook.com/stat_sim/histogram/index.html)
---
## Minimize AIC
* The Akaike Information Criterion is a popular model selection criterion
* Simple definition: $2k - 2 \log(\hat{L})$ where
- $k$ is the number of parameters (here the number of bins)
- $\hat{L}$ is the maximum likelihood (here the joint density under the histogram)
* Our prototype: make function of $k$ from input data
---
## AIC for histograms
```r
chooseBinsAIC <- function(x, p = 0.25)
{
x <- x[is.finite(x)]
n <- length(x)
r <- extendrange(x)
d <- r[2] - r[1]
histAIC <- function(B)
{
b <- seq(r[1], r[2], length.out = B + 1)
h <- hist(x, breaks = b, plot = FALSE)
## likelihood is simply product of density^counts
keep <- h$counts > 0 # skip 0 counts (by convention 0 log 0 == 0)
logL <- with(h, sum(counts[keep] * log(density[keep])))
2 * B - 2 * logL
}
Bvec <- seq(floor(sqrt(n)*p), ceiling(sqrt(n)/p))
list(range = r, B = Bvec,
AIC = unname(sapply(Bvec, histAIC)))
}
```
---
## AIC: implementation
```r
xx <- c(rnorm(500), rnorm(300, mean = 6, sd = 1.5))
ll <- chooseBinsAIC(xx, p = 0.125)
str(ll)
```
```
List of 3
$ range: num [1:2] -3.79 11.35
$ B : int [1:225] 3 4 5 6 7 8 9 10 11 12 ...
$ AIC : num [1:225] 4146 4115 3876 3736 3723 ...
```
---
## AIC: implementation
```r
with(ll, {
plot(AIC ~ B, type = "o")
abline(v = B[which.min(AIC)])
})
```
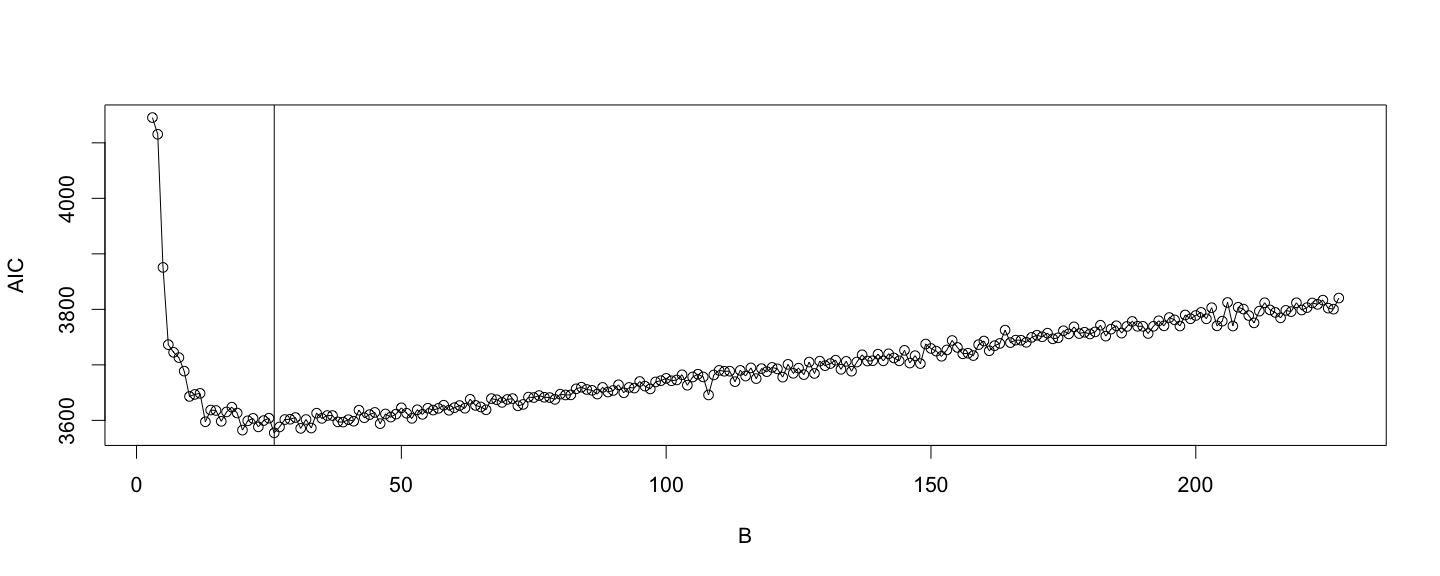
---
## AIC: comparison with other "rules"
```r
(nclass.aic <- with(ll, B[which.min(AIC)]))
```
```
[1] 26
```
```r
nclass.Sturges(xx)
```
```
[1] 11
```
```r
nclass.FD(xx)
```
```
[1] 12
```
```r
nclass.scott(xx)
```
```
[1] 12
```
---
## AIC: Resulting histogram
```r
breaks <- seq(ll$range[1], ll$range[2], length.out = nclass.aic + 1)
hist(xx, breaks = breaks)
```
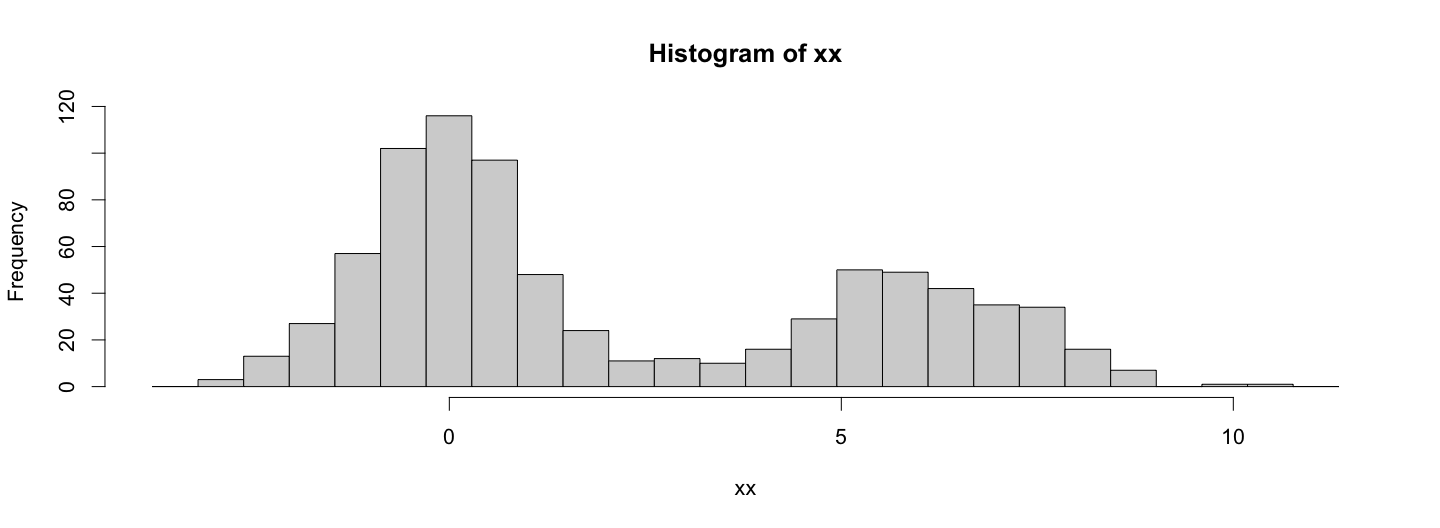
---
## Cross validation: summary
$\newcommand{\fhath}{\hat{f}_h}$
* Denote the histogram for bin width $h$ by $\fhath$, and the unknown density by $f$
* Want to choose $h$ to minimize integrated square error
$$
\int \left[ \fhath(x) - f(x) \right]^2 dx =
\int \fhath(x)^2 dx - 2 \int \fhath(x)\, f(x)\, dx + \int f(x)^2 dx
$$
* The third term is unknown but constant (as $h$ varies), so can be dropped
* The first term can be calculated from $\fhath$ (which is piecewise constant)
* The second term involves the unknown $f$, but the integral can be viewed as $E(\fhath(X))$ when $X \sim f$
---
## Cross validation: summary
* Leave-one-out estimator: average of ${\fhath}_{(-i)}(x_i)$
* The estimated integrated square error (without the constant term) simplifies to
$$
\frac{2}{(n-1) h} - \frac{n+1}{(n-1) n^2 h} \sum_{k} c_k^2
$$
* where $c_k$ are bin counts
---
## Cross validation: implementation
```r
chooseBins <- function(x, p = 0.25)
{
x <- x[is.finite(x)]
n <- length(x)
r <- extendrange(x)
d <- r[2] - r[1]
CVE <- function(B)
{
h <- d / B
breaks <- seq(r[1], r[2], length.out = B+1)
k <- findInterval(x, breaks) # runtime O(n * log(B))
counts <- table(factor(k, levels = 1:B))
2 / ((n-1)*h) - sum(counts^2) * (n+1) / ((n-1) * n^2 * h)
}
Bvec <- seq(floor(sqrt(n)*p), ceiling(sqrt(n)/p))
list(range = r, B = Bvec, CVE = unname(sapply(Bvec, CVE)))
}
```
---
## Cross validation: implementation
```r
## xx <- c(rnorm(500), rnorm(300, mean = 6, sd = 1.5))
ll <- chooseBins(xx, p = 0.125)
str(ll)
```
```
List of 3
$ range: num [1:2] -3.79 11.35
$ B : int [1:225] 3 4 5 6 7 8 9 10 11 12 ...
$ CVE : num [1:225] -0.0831 -0.0802 -0.1034 -0.1207 -0.1195 ...
```
---
## Cross validation: implementation
```r
with(ll, {
plot(CVE ~ B, type = "o")
abline(v = B[which.min(CVE)])
})
```
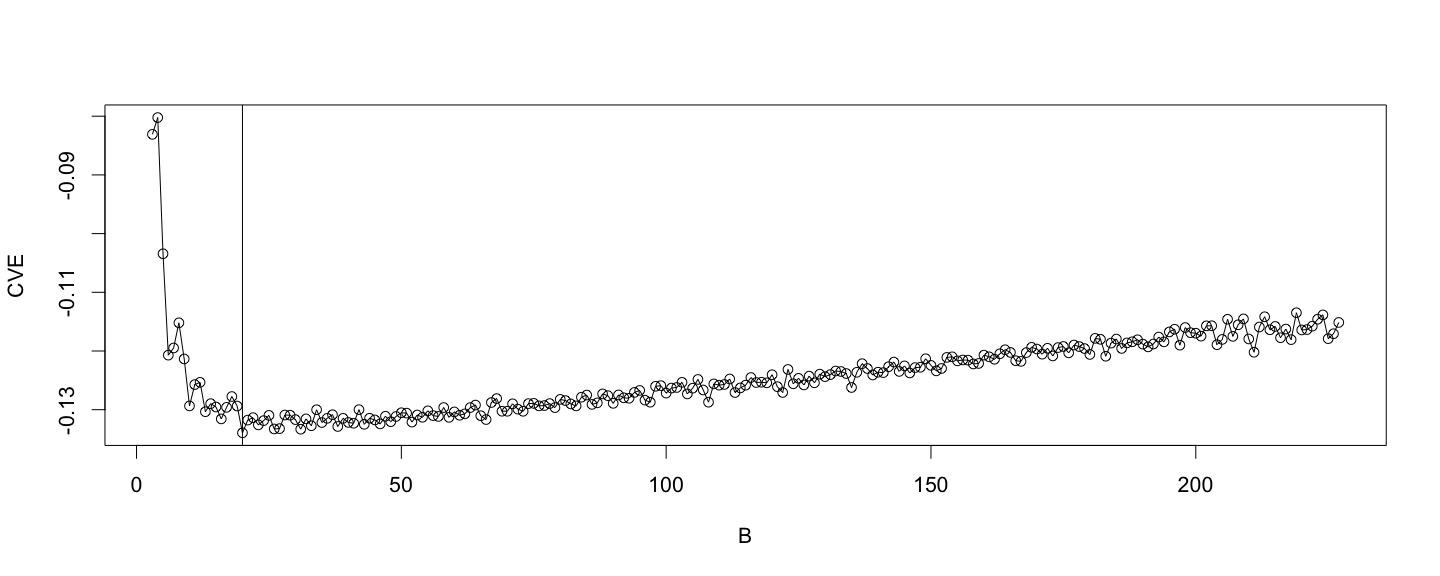
---
## Cross validation: comparison with other "rules"
```r
(nclass.cv <- with(ll, B[which.min(CVE)]))
```
```
[1] 20
```
```r
nclass.Sturges(xx)
```
```
[1] 11
```
```r
nclass.FD(xx)
```
```
[1] 12
```
```r
nclass.scott(xx)
```
```
[1] 12
```
---
## Cross validation: Resulting histogram
```r
breaks <- seq(ll$range[1], ll$range[2], length.out = nclass.cv + 1)
hist(xx, breaks = breaks)
```
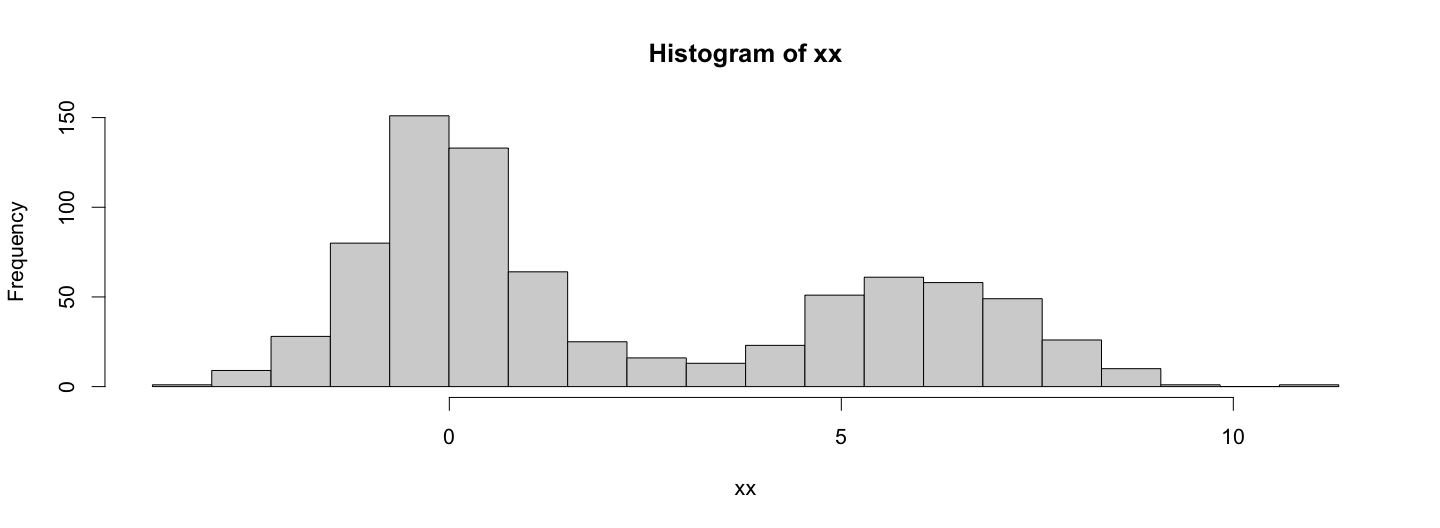
* For more interesting examples, see Gentleman and Ihaka,
[Lexical Scope and Statistical Computing](https://www.stat.auckland.ac.nz/~ihaka/downloads/lexical.pdf)
---
## Related topic: non-standard evaluation
* Again, consider an expression that will cause an error when evaluated
```r
rm(list = c("x", "y")) # remove x, y if defined earlier
plot(x, y, type = "l")
```
```
Error in plot(x, y, type = "l"): object 'x' not found
```
---
## Quoted (unevaluated) expressions
* R can actually work with an expression without evaluating it
```r
e <- quote(plot(x, y, type = "l"))
e
```
```
plot(x, y, type = "l")
```
```r
e[[1]]
```
```
plot
```
```r
e[[2]]
```
```
x
```
```r
e[[3]]
```
```
y
```
---
## Evaluating Quoted expressions
* A quoted expression can be evaluated using `eval()`
```r
eval(e)
```
```
Error in plot(x, y, type = "l"): object 'x' not found
```
* Fails because current environment (`.GlobalEnv`) does not contain variables `x` and `y`
* But Recall: Environment `e2` defined earlier contains
```r
ls.str(e2)
```
```
x : num [1:101] 0 0.0628 0.1257 0.1885 0.2513 ...
y : num [1:101] 1 0.998 0.992 0.982 0.969 ...
```
---
## Evaluating Quoted expressions
```r
eval(e, envir = e2)
```
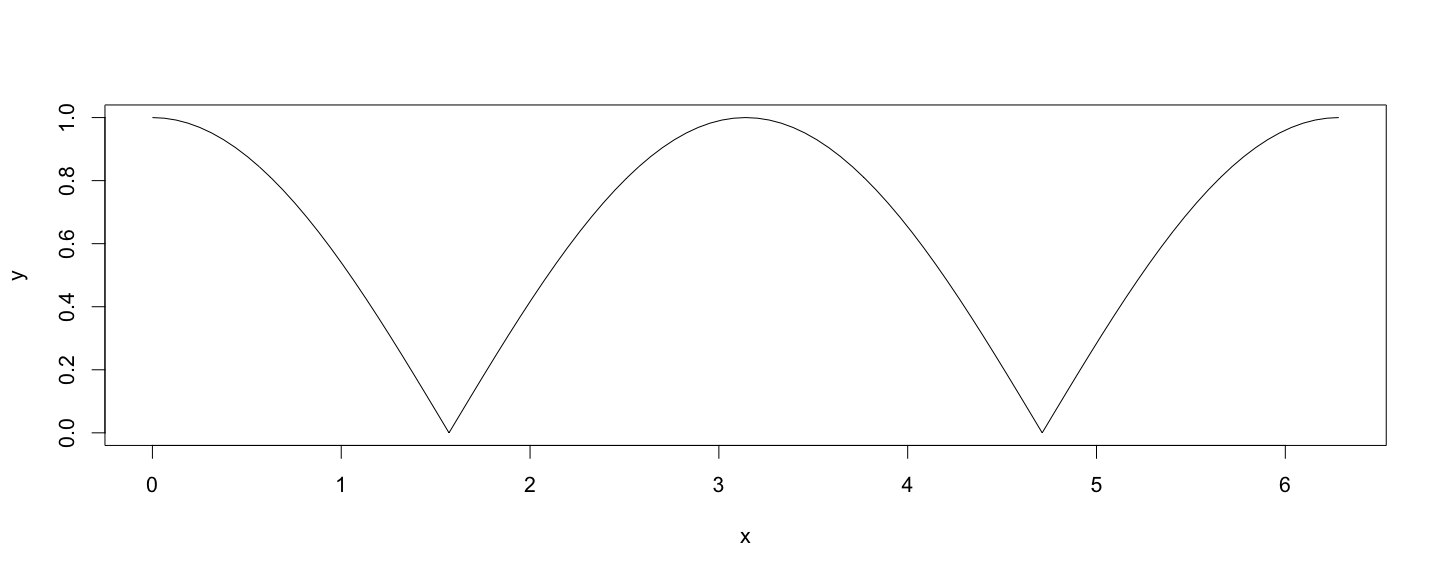
---
## Can we re-implement the `replicate()` function?
* This is how functions like `replicate()` and `with()` work
```r
my.replicate <- function(N, e)
{
ans <- numeric(N)
for (i in 1:N) ans[[i]] <- eval(e)
ans
}
```
* But we have to be careful to use it
```r
my.replicate(5, max(rnorm(10))) # not what we wanted
```
```
[1] 2.1574 2.1574 2.1574 2.1574 2.1574
```
```r
my.replicate(5, quote(max(rnorm(10))))
```
```
[1] 0.5284321 0.6858938 1.3573033 0.8895072 1.6434051
```
---
## Non-standard evaluation
* The `substitute()` trick: non-standard evaluation (using lazy argument evaluation)
```r
my.replicate <- function(N, e)
{
expr <- substitute(e) # avoids the need to quote()
ans <- numeric(N)
for (i in 1:N) ans[[i]] <- eval(expr)
ans
}
str(x <- my.replicate(1000, max(rnorm(20))))
```
```
num [1:1000] 1.648 2.524 1.636 1.346 0.729 ...
```
---
## Lazy evaluation
* Lazy evaluation: function arguments are only evaluated when needed
```r
set.seed(123)
rm(sqrt) # remove the sqrt() we defined earlier
chooseOne <- function(a, b, u = runif(1))
{
if (u < 0.5) a else b
}
```
* Only one of `a` and `b` is evaluated in any particular execution
```r
chooseOne(sqrt(2), sqrt(-2)) # no warning message
```
```
[1] 1.414214
```
```r
chooseOne(sqrt(2), sqrt(-2)) # warning message
```
```
Warning in sqrt(-2): NaNs produced
```
```
[1] NaN
```
---
## Further reading
```r
? environment
? eval
? expression
? quote
? bquote
? substitute
? with
```
```r
with.default
```
```
function (data, expr, ...)
eval(substitute(expr), data, enclos = parent.frame())
```
```r
replicate
```
```
function (n, expr, simplify = "array")
sapply(integer(n), eval.parent(substitute(function(...) expr)),
simplify = simplify)
```